
The swathe of tools from Google is designed to replace manual log-in and inventory-checking processes and relies on the intervention of AI in retail.
Shelf-checking AI helps retailers improve product availability
Google Cloud’s new AI-powered shelf-checking solution claims to help
retailers improve on-shelf product availability, provide better visibility into
what their shelves actually look like, and help them understand where restocks
are needed.
Built on Google Cloud’s Vertex AI Vision and powered by two machine learning
models—a product recogniser and tag recogniser—the shelf-checking AI enables
retailers to solve a very difficult problem: how to identify products of all
types, at scale, based solely on the visual and text features of a product, and
then translate that data into actionable insights.
“Retailers don’t have to expend time, effort, and investment into data
collection and training their own AI models,” said Google. “Leveraging Google’s database of billions of unique entities, Google Cloud’s shelf-checking AI can identify products from a variety of image types taken at different angles and vantage points—an especially difficult task.
Retailers will have a high degree of flexibility in the types of imagery
they can supply to the shelf-checking AI. For example, a retailer can use
imagery from a ceiling-mounted camera, an associate’s mobile phone, or a
store-roaming robot on shelf-checking duty.”
The project is in its pilot stage and is expected to be rolled out globally
in the coming months.
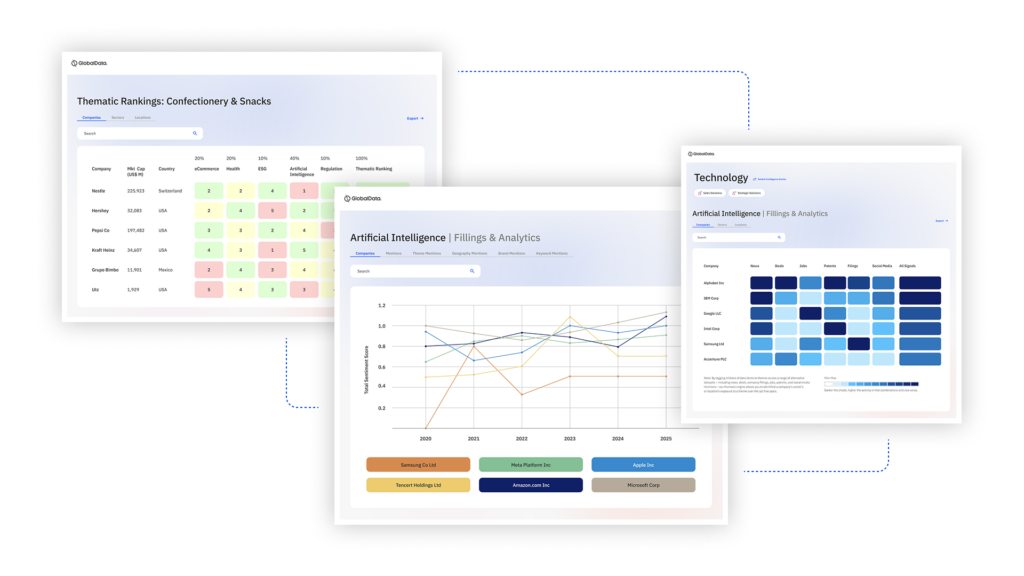
US Tariffs are shifting - will you react or anticipate?
Don’t let policy changes catch you off guard. Stay proactive with real-time data and expert analysis.
By GlobalDataTransforming digital window shopping experience with AI
To help retailers make the online browsing and product discovery experience
more modern, faster, intuitive, and fulfilling for shoppers, Google Cloud
introduced a new AI-powered browse feature in its Discovery AI solutions for retailers. The capability uses machine learning to select the optimal ordering of products on a retailer’s e-commerce site once shoppers choose a category, like
“women’s jackets” or “kitchenware.”
Over time, the AI learns the ideal product ordering for each page on an
e-commerce site using historical data, optimising how and what products are
shown for accuracy, relevance, and the likelihood of making a sale. The feature
can be used on a variety of e-commerce site pages, from browse, brand, and
landing pages, to navigation and collection pages.
Machine learning enabled personalised search and browsing
To help retailers create more fluid and intuitive online shopping
experiences, Google Cloud has introduced a new AI-driven personalisation
capability that customises the results a customer gets when they search and browse
a retailer’s website. The technology turbo-charges the capabilities of Google
Cloud’s new browse offering and existing Retail Search solution.
The AI underpinning the new personalisation capability is a product-pattern
recogniser that uses a customer’s behaviour on an e-commerce site, such as
their clicks, cart, purchases, and other information, to determine shopper
taste and preferences. The AI then moves products that match those preferences
up in search and browse rankings for a personalised result.
AI increases retailers’ bottom line with better recommendations
Google Cloud’s Recommendations AI solution uses machine learning to help
retailers bring product recommendations to their shoppers.
New upgrades can make a retailer’s e-commerce properties even more personalised, dynamic and helpful for individual customers. For example, a new page-level optimisation feature now enables an e-commerce site to dynamically decide what product recommendation panels to uniquely show to a shopper. Page-level optimisation also minimises the need for resource-intensive user experience testing, and can improve user engagement and conversion rates.
In addition, a recently added revenue optimisation feature uses machine
learning to offer better product recommendations that can lift revenue per user
session on any e-commerce site. A machine learning model, built-in
collaboration with DeepMind, combines an ecommerce site’s product
categories, item prices, and customer clicks and conversions to find the right
balance between long-term satisfaction for shoppers and revenue lift for
retailers. Finally, a new buy-it-again model leverages a customer’s shopping
history to provide personalised recommendations for potential repeat purchases.